Organized by
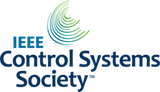 |
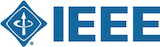 |
|
Technically co-sponsored by |
|
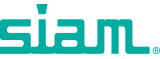 |
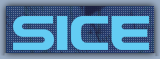 |
Your IP: 216.73.216.242
Unique visitors: 163008
Total Page views: 28799 (Since February 1st,
2018)
Contact the webmaster
Credits photo: Office de Tourisme de Nice, France
Credits logo: Tania Canudas de Wit
|
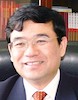 |
Bode Lecture: Lei Guo
Chinese Academy of Sciences, China
Feedback and Uncertainty: Some Basic Problems and Theorems
Time and location: 8:30-9:30, Friday, December 13, 2019 - Apollon
Slides available
Abstract: Feedback is a core concept of automatic control, a fundamental principle of systems and an indispensable mechanism in intelligent systems, which makes it possible for a dynamical system to perform well in the presence of various uncertainties. Although it is widely recognized that a comprehensive investigation of the quantitative relationship between feedback and uncertainty is a challenging task, considerable progress has been made in both theory and practice on the design and analysis of feedback systems. In this lecture, we will present some findings and theorems in the understanding of several basic problems. First, we will consider adaptive control of linear stochastic systems and explain the difficulties and techniques in establishing the global stability and optimality of the well-known self-tuning regulators (STR), designed by combining the least-squares estimator with the minimum variance controller. This natural and seemingly simple case had actually been a basic longstanding open problem in adaptive control, and its solution offers valuable insights necessary for more complicated problems. Next, we will discuss the theoretical foundation of the classical proportional-integral-derivative (PID) control, to understand the rationale behind its widespread successful applications in control practice where almost all of the systems are nonlinear with uncertainty, by presenting some theorems on the global (semi-global) stability and asymptotic optimality of the closed-loop systems, and by providing a concrete design method for the PID parameters. Finally, we will consider more fundamental problems on the maximum capability and limitations of the feedback mechanism in dealing with uncertain nonlinear systems, where the feedback mechanism is defined as the class of all possible feedback laws (which are not restricted to a certain particular subclass). We will present some “critical values” and “impossibility theorems” about the maximum capability of the feedback mechanism for several basic classes of uncertain nonlinear systems. Experiences, extensions and expectations will also be shared during the lecture.
Biography: Lei Guo received his B.S. degree in mathematics from Shandong University in 1982, and Ph.D. degree in control theory from the Chinese Academy of Sciences in 1987. He was a postdoctoral fellow at the Australian National University (1987-1989). Since 1992, he has been a Professor of the Institute of Systems Science at the Chinese Academy of Sciences (CAS). From 2002 to 2012,he was the President of the Academy of Mathematics and Systems Science, CAS. He is currently the Director of the National Center for Mathematics and Interdisciplinary Sciences, CAS. He has worked on problems in adaptive control, system identification, adaptive signal processing, and stochastic systems. His current research interests include control of nonlinear uncertain systems, PID control theory, distributed filtering and estimation, capability of feedback, multi-agent systems, game-based control systems, and complex systems, among others.
|
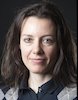 |
Domitilla Del Vecchio
Massachusetts Institute of Technology , USA
Genetic Circuit Engineering Meets Control Theory
Time and location: 8:30-9:30, Wednesday, December 11, 2019 - Apollon
Slides available
Abstract: Genetic circuits control every aspect of life and thus the ability to engineer them de-novo opens exciting possibilities, from revolutionary drugs and green energy, to bugs that recognize and kill cancer cells. The robustness of natural gene networks is the result of million years of evolution and is in contrast with the fragility of today’s engineered circuits. A genetic module’s input/output behavior changes in unpredictable ways upon inclusion into a larger system. Therefore, each component of a system is usually redesigned every time a new piece is added. Rather than relying on such ad-hoc design procedures, control theoretic approaches may be used to engineer “insulation” of circuit components from context, thus enabling modular composition through specified input/output connections. In this talk, I will give an overview of modularity failures in genetic circuits, focusing on problems of loads, and introduce a control-theoretic framework, founded on the concept of retroactivity, to address the insulation question. Within this framework, insulation can be mathematically formulated as a disturbance rejection problem; however, classical solutions are not directly applicable due to bio-physical constraints. I will thus introduce solutions relying on time-scale separation, a key property of biomolecular systems, which we used to build two devices: the load driver and the resource decoupler. These devices aid modularity, facilitate predictable composition of genetic circuits, and show that control theoretic approaches may be suitable to address pressing challenges in engineering biology.
Biography:
Domitilla Del Vecchio received the Ph. D. degree in Control and Dynamical Systems from the California Institute of Technology, Pasadena, and the Laurea degree in Electrical Engineering (Automation) from the University of Rome at Tor Vergata in 2005 and 1999, respectively. From 2006 to 2010, she was an Assistant Professor in the Department of Electrical Engineering and Computer Science and in the Center for Computational Medicine and Bioinformatics at the University of Michigan, Ann Arbor. In 2010, she joined Department of Mechanical Engineering at the Massachusetts Institute of Technology (MIT), where she is currently Professor and member of the Synthetic Biology Center. She is a recipient of the 2016 Bose Research Award (MIT), the Donald P. Eckman Award from the American Automatic Control Council (2010), the NSF Career Award (2007), the American Control Conference Best Student Paper Award (2004), and the Bank of Italy Fellowship (2000).
|
|
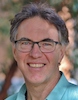 |
Robert Mahony
Australian National University, Australia
Equivariant Observers: Robust nonlinear state estimation for
robotic systems
Time and location: 8:30-9:30, Wednesday, December 11, 2019 - Athena
Slides available
Abstract: The physical state of a robotic system naturally carries
structure; the pose of rigid links can be written as elements of
the Special Euclidean group, images taken by a camera of a planar
scene can be related by homographies and mapped to elements of the
special linear group, etc. Recent work has demonstrated that there
is a rich collection of symmetry groups for different robotic
problems above and beyond the classical Lie-groups. This talks
shows how this structure can be exploited to design robust
nonlinear observers for state estimation. The earliest results in
this direction were nonlinear attitude estimators (2005-2010) that
were an enabling technology in the aerial robotic vehicle industry.
Pose estimation algorithms based on these ideas are built into the
augmented reality headsets that are now ubiquitous in gaming.
Recent symmetries have opened the door to new solutions for
classical robotics problems such as visual odometry, visual
inertial odometry, simultaneous localisation and mapping.
Biography:
Robert Mahony is a Professor in the Research School of Engineering at the
Australian National University. He received his BSc in 1989 (applied mathematics and geology) and his PhD in 1995 (systems engineering) both from
the Australian National University. He is a fellow of the IEEE and was
president of the Australian Robotics Association from 2008-2011. He was
Director of the Research School of Engineering at the Australian National
University 2014-2016. His research interests are in non-linear systems
theory with applications in robotics and computer vision. He is known for his
work in aerial robotics, equivariant observer design, matrix subspace
optimisation and image based visual servo control.
|
|
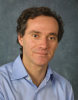 |
Alessandro Astolfi
Imperial College, UK and Univ. Rome Tor Vergata, Italy
The curse of linearity and time-invariance
Time and location: 8:30-9:30, Thursday, December 12, 2019 - Athena
Slides available
Abstract: The study of linear systems theory without exploiting linearity and time-invariance may pose challenges, yet it is highly rewarding. In truth, linearity and time-invariance, albeit powerful, are a curse: they are not conducive to an abstract understanding of concepts, tools and ideas and may often be misleading.
On the other hand, notions such as manifold invariance, interconnection, coordinates transformations, decomposition, and the principle of optimality facilitate the enhancement of linear, time-invariant, systems theory methods and tools to far more general classes of systems.
We illustrate this perspective by providing abstract and geometric definitions for eigenvalues, poles, moments, Loewner operators and derivative, and persistence of excitation; and by solving interpolation problems, adaptive and robust control problems, and optimal control and game theory problems, for general classes of nonlinear systems.
Biography: Alessandro Astolfi was born in Rome, Italy, in 1967. He graduated in electrical engineering from the University of Rome in 1991. In 1992 he joined ETH-Zurich where he obtained a M.Sc. in Information Theory in 1995 and the Ph.D. degree with Medal of Honor in 1995 with a thesis on discontinuous stabilisation of nonholonomic systems. In 1996 he was awarded a Ph.D. from the University of Rome "La Sapienza" for his work on nonlinear robust control. Since 1996 he has been with the Electrical and Electronic Engineering Department of Imperial College London, London (UK), where he is currently Professor of Nonlinear Control Theory and Head of the Control and Power Group. From 1998 to 2003 he was also an Associate Professor at the Dept. of Electronics and Information of the Politecnico of Milano. Since 2005 he has also been a Professor at Dipartimento di Ingegneria Civile e Ingegneria Informatica, University of Rome Tor Vergata. His research interests are focussed on mathematical control theory and control applications, with special emphasis for the problems of discontinuous stabilisation, robust and adaptive control, observer design and model reduction.
|
|
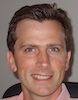 |
Francis Bach
INRIA, France
Distributed Machine Learning over Networks
Time and location: 8:30-9:30, Thursday, December 12, 2019 - Apollon
Slides available
Abstract:
Abstract: The success of machine learning models is in part due to their capacity to train on large amounts of data. Distributed systems are the common way to process more data than one computer can store, but they can also be used to increase the pace at which models are trained by splitting the work among many computing nodes. In this talk, I will study the corresponding problem of minimizing a sum of functions which are respectively accessible by separate nodes in a network. New centralized and decentralized algorithms will be presented, together with their convergence guarantees in deterministic and stochastic convex settings, leading to optimal algorithms for this particular class of distributed optimization problems.
Biography: Francis Bach is a researcher at INRIA, leading since 2011 the SIERRA project-team, which is part of the Computer Science Department at Ecole Normale Supérieure, and a joint team between CNRS, ENS and INRIA. Since 2016, he is an adjunct Professor at Ecole Normale Supérieure. He completed his Ph.D. in Computer Science at U.C. Berkeley, working with Professor Michael Jordan, and spent two years in the Mathematical Morphology group at Ecole des Mines de Paris, he then joined the WILLOW project-team at INRIA/Ecole Normale Superieure/CNRS from 2007 to 2010. He obtained in 2009 a Starting Grant and in 2016 a Consolidator Grant from the European Research Council, and received the Inria young researcher prize in 2012, the ICML test-of-time award in 2014, as well as the Lagrange prize in continuous optimization in 2018. In 2015, he was program co-chair of the International Conference in Machine learning (ICML), and general chair in 2018; he is now co-editor-in-chief of the Journal of Machine Learning Research. Francis Bach is primarily interested in machine learning, and especially in graphical models, sparse methods, kernel-based learning, large-scale convex optimization, computer vision and signal processing.
|
|
Key dates (2019)
Submission Site Open: | January 4 |
Initial Paper Submissions to L-CSS with CDC Option Due: | March 1 |
Invited Session Proposals Due: | March 7 |
Initial Paper Submissions Due: | March 17 |
Tutorial Session Proposals Due: | March 31 |
Workshop Proposals Due: | May 2 |
Paper and Workshop Decision Notification: | mid-July |
Final Submission Open: | August 1 |
Registration Opens: | August 1 |
Best Student Paper Nominations Opens: | August 1 |
Best Student Paper Nominations Closes: | August 15 |
Accepted Papers Due: | September 12 |
Early Bird Closes: | October 1 |
Conference opens: | December 11 |
Conference closes: | December 13 |
Gold Sponsors
Silver Sponsors
Bronze Sponsors
Copper Sponsors
Click here to see the complete list of sponsors and exhibitors
|